Using Math to Study Ecology
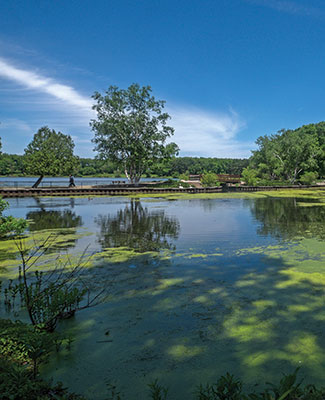
Using Math to Study Ecology
January 26, 2016Understanding the overall structures of ecological communities is crucial to maintaining the health of ecosystems. One such complex but vital community is the plankton in freshwater lakes. Combining mathematical models with experiments and analysis of field data, two MSU professors are working to discover the causes behind some of ecology’s most widespread structural patterns by studying the structure of plankton communities in lakes and oceans.
The work has received support from the Simons Foundation of New York, which has awarded its first major grant to Michigan State University to support the work of Drs. Christopher Klausmeier and Elena Litchman to study how interactions between species scale up to determine why most species are rare and only a few common in any community. The study is entitled “Microscopic Foundations For Macroecological Patterns,” a project fitting the Simons Foundation’s mission to advance the frontiers of research in mathematics and the basic sciences.
The Simons Foundation awarded the grant as part of its Targeted Grants in the Mathematical Modeling of Living Systems (MMLS) program, part of the foundation’s Mathematics and Physical Sciences and Life Sciences divisions.
The professors are based at MSU’s Kellogg Biological Station near Kalamazoo in southwest Michigan. Dr. Klausmeier is a theoretical ecologist who uses mathematical and computational models to uncover the principles that form the structure of ecological communities. His main focus is on plankton communities found in lakes and the ocean. Dr. Litchman is an experimental and conceptual ecologist who also focuses on phytoplankton communities. Her work applies trait-based approaches to plankton ecology, combining lab experiments with meta-analyses and modeling.
Species Abundance Distributions
Dr. Klausmeier explained that species abundance distributions (SADs) — the patterns of commonness and rarity in a community — have fascinated ecologists for over a century. “Over time many explanations have been developed, but they are often based on unrealistic biological assumptions or are merely statistical in nature. In addition, these existing explanations have been tested only by comparing patterns in nature with model predictions. The problem is that many models can lead to the same patterns, leaving these tests unable to distinguish among competing hypotheses.”
In the new study, Klausmeier and Litchman will develop new models based on more realistic assumptions of how species interact. One key is embedding a local community in the broader landscape through immigration of individuals. Another novel aspect of the models will be investigating the dynamics of these SADs in changing environments, such as the Michigan lakes the team studies. Finally, the team will put their new models to the test using controlled experiments, a more powerful form of model testing than has been applied to SAD theories.
Litchman and Klausmeier are involved in other ongoing projects, such as: (1) developing and testing models of the vertical distribution of phytoplankton; (2) understanding the role of spatial heterogeneity on species competition and coexistence; (3) determining the causes of seasonal succession in plankton communities; (4) applying game theoretical approaches to models of community assembly; (5) explaining different sources of variation in the elemental stoichiometry of phytoplankton; and (6) exploring the dynamics of nonlinear food web modules.
The Simons Foundation
The Simons Foundation’s MMLS program seeks to foster a culture of theory-experiment collaboration similar to the work in the physical sciences. It supports the development of mathematical models that explain classes of experimental results and suggest new directions for research and experimentation aimed at testing theoretical ideas and expanding their reach.
The Targeted Grant in MMLS program supports research in the life sciences that breaks new conceptual or theoretical ground and relates closely to experiment, for example, by introducing new and experimentally testable concepts or by developing models that can explain data and motivate new classes of experiments.